Researches on Sleep Staging based on Multivariate Multimodal Signals
Advisor: Prof. Youfang Lin, July 2020 - October 2021
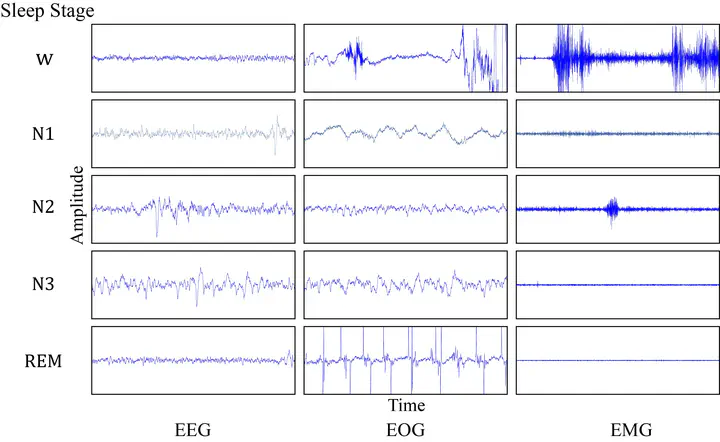
BrainSleepNet: Learning Multivariate EEG Representation for Automatic Sleep Staging
Designed a method for constructing temporal-spectral-spatial representation of EEG signals for describing different sleep stages from different perspectives
Fused the time-domain, frequency-domain, and spatial-domain features of EEG signals simultaneously in a unified network for learning comprehensive features of multivariate signals.
Published a co-first-authored paper on 2020 IEEE International Conference on Bioinformatics and Biomedicine.
SleepPrintNet: A Multivariate Multimodal Neural Network Based on Physiological Time-Series for Automatic Sleep Staging
- Introduced multimodal signals including EOG and EMG in addition to EEG for sleep staging.
- Developed modal-independent signal processing components for capturing discriminative features from each modality.
- Published a co-first-authored paper on IEEE Transactions on Artificial Intelligence.
Multi-modal Physiological Signals based Two-Stream Squeeze-and-Excitation Network for Sleep Staging
- Captured the heterogeneity of EEG and EOG signals by the multi-modal physiological signals representation and independent feature extraction networks.
- Adaptively utilized the features of EEG and EOG signals by a multi-modal Squeeze-and-Excitation feature fusion module for classification.
- Submitted a first-authored paper to IEEE Sensors Journal (in minor revision).